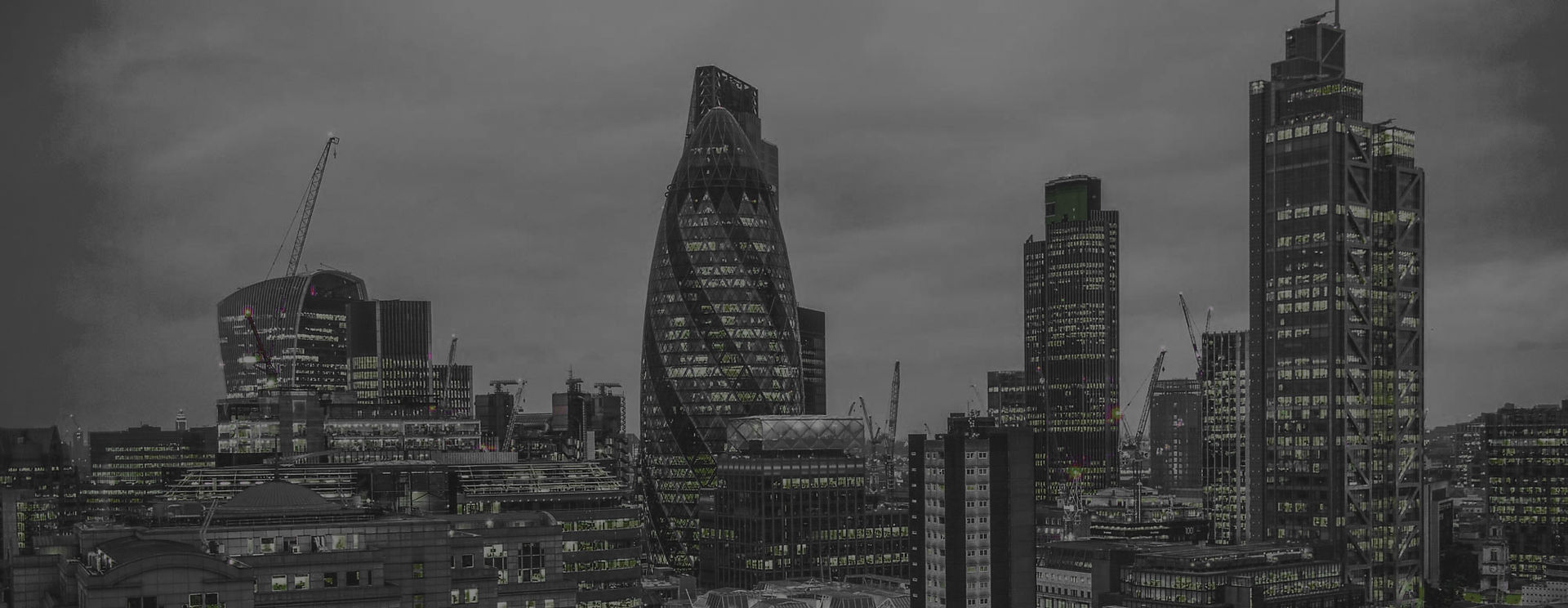
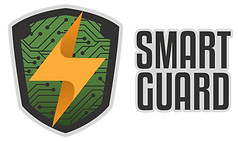

SMART GUARD
Deep Learning Detection of
Cyber-Attacks in Smart Grids
Team 4 Senior Design
To learn more about our project
OUR PROJECT
A movement towards smarter and more reliable systems.
Smart Guard focuses on enhancing Smart Grids into a more secured system by using Deep Machine-Learning. As Smart Grids allow bidirectional flow of information between the utility company and the customer, security issues arise making the system vulnerable for cyber-attacks. By the end of the project, the model will be capable of detecting False Data Injection (FDA), a type of attack that can lead to electricity theft and destruction of the power grid.
​
The project is implemented through two stages: training phase and testing phase. The model is fed a set of real power consumption data in order for it to understand the behavior of the customers. The training set also contains recordings manipulated by malicious attacks to enhance the learning process. Choosing deep Recurrent Neural Network as the model's algorithm allows it to be smarter than existing models. The parameters and hyper parameters of Smart Guard are optimized to create the highest Detection Rate and lowest False Alarm Rate. As the model proceeds into the second stage, testing
​
OUR TEAM
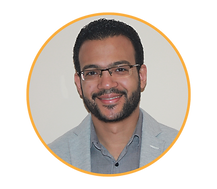
Dr. Muhammad Ismail
Project's Mentor
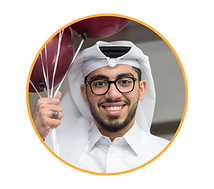
Ali Ali
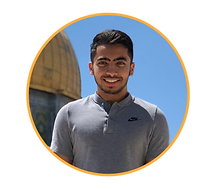
Karam Abulrub
Amir Rezk

Mowad Alaradi
Communication and
Documentation
Research and Data
Team Leader
Website Administrator
GET IN TOUCH
Texas A&M University at Qatar.